Industry Keynote I: AI in Multimedia Advertising — Wei Liu (Tencent, China)
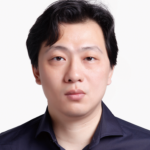
Speaker: Dr. Wei Liu is currently a Distinguished Scientist of Tencent, China. He takes the director of Ads Multimedia AI at Tencent Data Platform, and has taken the director of Computer Vision at Tencent AI Lab. Prior to joining Tencent, he received the Ph.D. degree in EECS from Columbia University, USA, and was a research scientist and staff member of IBM T. J. Watson Research Center, USA. Dr. Liu has long been devoted to fundamental research and technology development in core fields of AI. His research works win numerous awards and honors, e.g., the 2011 Facebook Fellowship, the 2012 IBM Josef Raviv Memorial Postdoctoral Fellowship, the 2013 Jury Award for Best Thesis of Columbia University, the 2016 and 2017 SIGIR Best Paper Award Honorable Mentions, the 2018 “AI’s 10 To Watch” Award, the 2020-2021 World’s Top 2% Scientists honor, etc. Dr. Liu is an Associate/Action Editor of internationally leading AI journals IEEE Transactions on Pattern Analysis and Machine Intelligence (TPAMI), IEEE Transactions on Neural Networks and Learning Systems (TNNLS), IEEE Intelligent Systems, and Transactions on Machine Learning Research (TMLR), and is an Area Chair of top-tier computer science and AI conferences NeurIPS, ICML, CVPR, ICCV, IJCAI, and AAAI. Dr. Liu is a Fellow of the IAPR (International Association for Pattern Recognition), AAIA (Asia-Pacific Artificial Intelligence Association), IMA (Institute of Mathematics and its Applications), BCS (British Computer Society), and RSA (Royal Society of Arts), and is an Elected Member of the ISI (International Statistical Institute).
Abstract: In this talk, I plan to present core AI problems that contemporary online advertising in China encounters. Multimedia contents are widespread in Chinese Internet advertisements, which makes us to research and develop powerful deep learning techniques to understand, generate, and distribute those contents in a more precise, high-fidelity, and rapid manner. Our developed deep learning techniques include image/video classification, image/video captioning, image/video hashing, video segmentation and summarization, cross-modal retrieval, image segmentation and inpainting, 3D estimation from image, neural rendering, etc.
Industry Keynote 2: Trustworthy Machine Learning — Kush R Varshney (IBM, US)
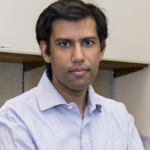
Speaker: Kush R. Varshney was born in Syracuse, NY in 1982. He received the B.S. degree (magna cum laude) in electrical and computer engineering with honors from Cornell University, Ithaca, NY, in 2004. He received the S.M. degree in 2006 and the Ph.D. degree in 2010, both in electrical engineering and computer science from the Massachusetts Institute of Technology (MIT), Cambridge. While at MIT, he was a National Science Foundation Graduate Research Fellow. Dr. Varshney is a distinguished research scientist and manager with IBM Research at the Thomas J. Watson Research Center, Yorktown Heights, NY, where he leads the machine learning group in the Foundations of Trustworthy AI department. He was a visiting scientist at IBM Research – Africa, Nairobi, Kenya in 2019. He is the founding co-director of the IBM Science for Social Good initiative. He applies data science and predictive analytics to human capital management, healthcare, olfaction, computational creativity, public affairs, international development, and algorithmic fairness, which has led to recognitions such as the Gerstner Award for Client Excellence for contributions to the WellPoint team, the Extraordinary IBM Research Technical Accomplishment for contributions to workforce innovation and enterprise transformation, and Falling Walls Science and Innovation finalist for AI Fairness 360. He conducts academic research on the theory and methods of trustworthy machine learning. His work has been recognized through best paper awards at the Fusion 2009, SOLI 2013, KDD 2014, and SDM 2015 conferences and the 2019 Computing Community Consortium / Schmidt Futures Computer Science for Social Good White Paper Competition. He published the book entitled ‘Trustworthy Machine Learning’ (http://www.trustworthymachinelearning.com/) in 2022. He is a senior member of the IEEE.
Abstract: We will discuss the concepts for developing accurate, fair, robust, explainable, transparent, inclusive, empowering, and beneficial machine learning systems. Accuracy is not enough when you’re developing machine learning systems for consequential application domains. You also need to make sure that your models are fair, have not been tampered with, will not fall apart in different conditions, and can be understood by people. Your design and development process has to be transparent and inclusive. You don’t want the systems you create to be harmful, but to help people flourish in ways they consent to. All of these considerations beyond accuracy that make machine learning safe, responsible, and worthy of our trust have been described by many experts as the biggest challenge of the next five years.